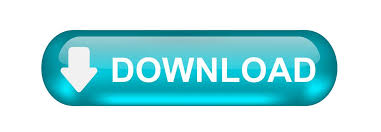
A network visualization analysis showed that the DNN automatically detected features from areas of the brain related to each task. The proposed method achieved an average accuracy of 89.0 and 94.7% on a working memory task and a motor classification task, respectively, higher than the accuracy of 69.2 and 68.6% obtained by the SVM-MVPA. We also showed the general applicability of the DNN for transfer learning to small datasets (N = 43), a situation encountered in typical neuroscience research. In tests to verify its performance, the proposed classification method identified seven tasks with an average accuracy of 93.7%. We trained and tested the DNN classifier using task fMRI data from the Human Connectome Project's S1200 dataset (N = 1,034). In this study, we propose a deep neural network (DNN) for directly decoding multiple brain task states from fMRI signals of the brain without any burden for feature handcrafts. Conventionally, the SVM-MVPA requires careful feature selection/extraction according to expert knowledge. Vision Research, 41, 3751 – 3761.Support vector machine (SVM)-based multivariate pattern analysis (MVPA) has delivered promising performance in decoding specific task states based on functional magnetic resonance imaging (fMRI) of the human brain. (2001) Non-veridical visual motion perception immediately after saccades. (2007) Spatial attention and the latency of neuronal responses in macaque area V4. Lee, J., Williford, T., & Maunsell, J.H.R. (2009) A normalization model of attentional modulation of single unit responses. Journal of Neurophysiology, 104, 960 – 971. (2010) The effect of attention on neuronal responses to high and low contrast stimuli. (2010) Attentional modulation of MT neurons with single or multiple stimuli in their receptive fields. (2012) The interaction of bayesian priors and sensory data and its neural circuit implementation in visually guided movement. (2013) Gamma synchrony predicts neuron–neuron correlations and correlations with motor behavior in extrastriate visual area MT. (2013) Control of the gain of visual–motor transmission occurs in visual coordinates for smooth pursuit eye movements. (2016) Signal, noise, and variation in neural and sensory-motor latency. § Lee, J., Joshua, M., Medina, J.F., & Lisberger, S.G. (2019) Motion direction representation in multivariate electroencephalography activity for smooth pursuit eye movements. Frontiers in Systems Neuroscience, 13 71. (2019) Effect of prior direction expectation on the accuracy and precision of smooth pursuit eye movements. (2020) Predicting Trial-By-Trial Variation in Oculomotor Behavior Using Multivariate Electroencephalography Theta Phase. (2020) The neural basis for response latency in a sensory-motor behavior. Lee, J., Darlington, T.R., & Lisberger, S.G. (2021) Induced astigmatism biases the orientation information represented in multivariate electroencephalogram activities.
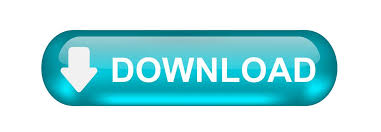